Organisations do not suffer from a lack of data or qualified people who analyse it and draw conclusions but rather from the size of relevant data sets and the limited amount of time skilled people can spend on exploration and analysis. In this blog post we show how Hume Alerts can be used to solve this issue.
But before we get started, I would like to take you to a very analog place, where, even without high speed internet, Alerts work.
It is a sunny morning in early April in the North of Sweden, the air is cold and crisp. As I step out of the small cabin in the woods where I used to spend my weekends, I am mesmerized by the thin layer of snow that has come down overnight and now covers the trees, the meadows and the veranda. The veranda… There are paw prints. Lots of them. In the thin snow layer on top of the wooden floor.
In my hand I hold the leftovers of the apples I had for breakfast, I reach for the compost bag that I keep safely stored on a chair outside during nights – there are mice in the forest and I do not want to give them a reason to come inside the cabin. My hand grabs into the empty. No compost bag. I look at the chair, scan it all the way down to its feet. Pawprints. And no compost bag… My gaze returns to the floor: the pawprints come from the meadow, make their way onto the veranda, towards the chair and there they turn around. There is another track right next to them. As if something was dragged along. I follow the prints out into the meadow. After a few meters, I find some avocado peels and carrot skins in the snow. After about fifty meters, I see the brown compost bag ripped open, its contents splattered around the meadow.
After cleaning up the mess, I get the measuring tape and return to the pawprint: it is about four to five centimeters long and evenly formed, the digital pads gather around the metacarpal pad in perfect symmetry – almost like a flower with even petals. Small imprints of claws are visible on top of the digital pads. I check my app: all points towards a fox. I have seen a fox around many times. The compost bag has also disappeared and resurfaced in a torn and empty state all over the place many times. I have never been able to connect the two dots. Until the snow came.
In this case, the snow acted as an alert that raised my attention to something that was there, but previously not visible to me. I needed the snow-alert to see the evidence and relation between two points – the fox and the compost bag. The insight I gained through exploring the data (which animal is it) enables me to store the compost bag in a place where neither mice nor the fox can reach it.
Outside the forest, there is other data that seems unconnected and seemingly unrelated to the problems we want to solve. Often, the datasets are so big and the occurrence of evidence so unpredictable that we simply are at a loss when deciding where to start an analysis.
Alerts in law enforcement
Law enforcement agencies often know which persons represent a potential risk to society. They are able to gather information on these individuals and, thanks to graphs, on the relationships that these people have and on activities they are involved in. But how can they know when the person acquires a new, potentially dangerous relationship, let’s say with another individual of interest? Or when the person conducts a suspicious transaction with their credit card? We all know the scenes from spy movies: an agent shadows the suspect day and night and notes down all movements and meetings. Luckily, today our law enforcement units do not have to rely solely on manual data gathering.
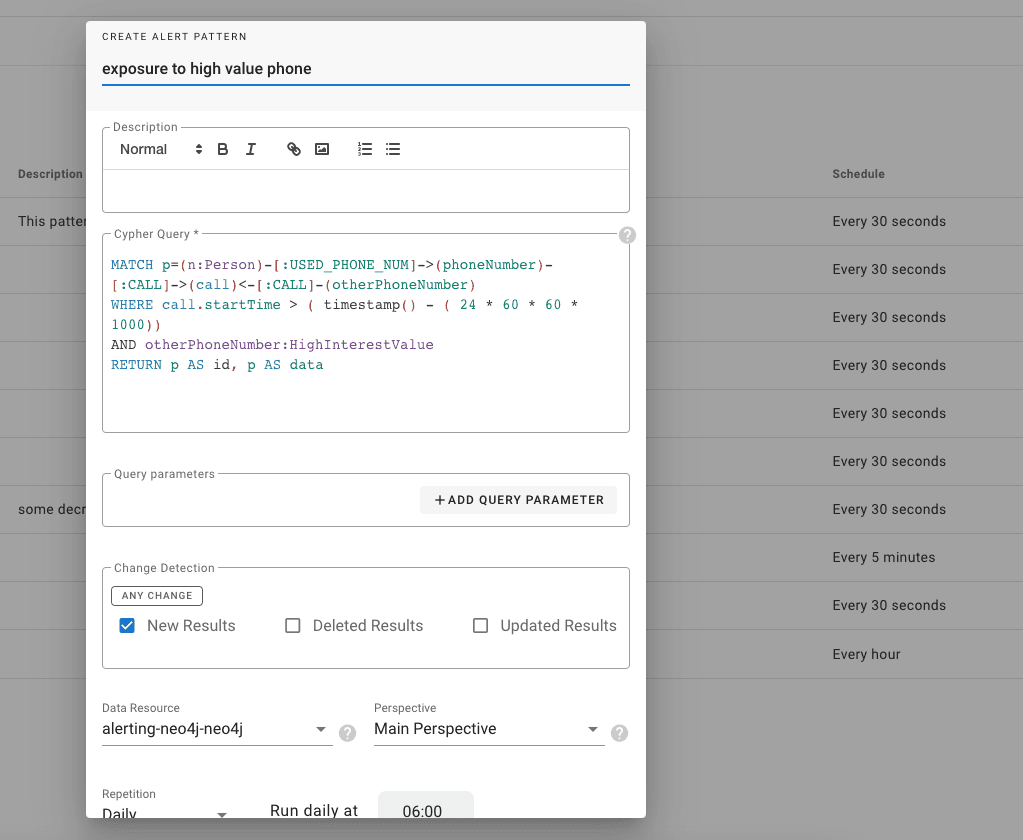
As we saw in the beginning of June with Operation Ironside, technology is the driving force behind crime prevention and law enforcement. Capabilities to gather data, decrypt and read encrypted communication are well developed. Traps can be built like the communications app ANOM that contributed massive amounts of data to Operation Ironside. Now that the data is secured, it needs to be analysed – and here time is of essence.
Analysts at law enforcement agencies know which questions to explore in the huge dataset. The issue is that data is subject to permanent change and it is sheerly impossible to monitor all relevant data manually, without the support of alerts.
With Hume Alerts, questions can be formulated in the form of a pattern. Thanks to Cypher, it is easy to cover any type of question and regularly comb the database for relevant changes in the data. An alert uses patterns and connects them to entities of interest, sharpening the focus of the question. Automated notifications offload analysts from the burden of proactively checking for changes. Analysts can now spend more time on exploration and deduction of insights, while alerts keep them up to date.
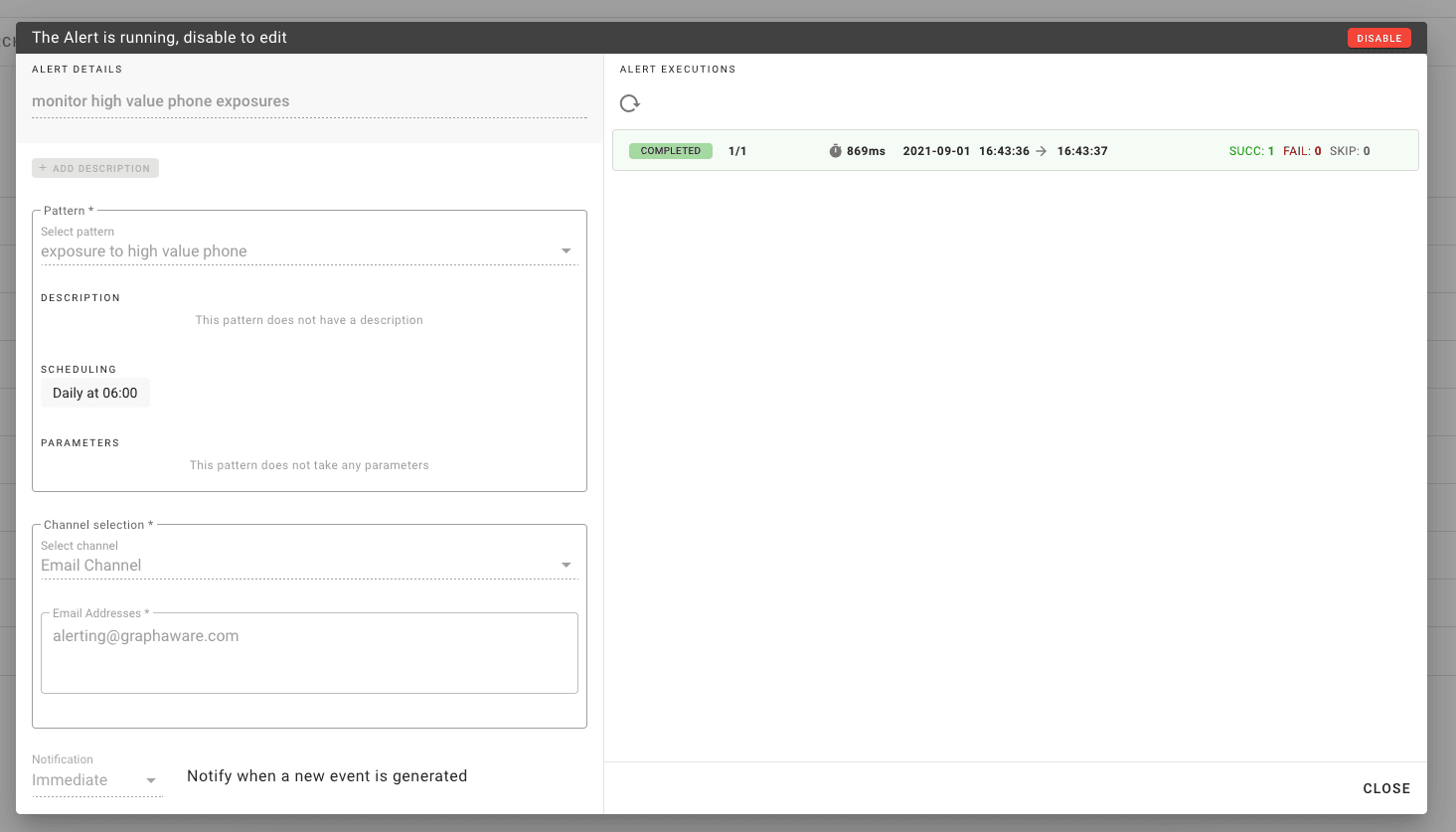
Alerts in Life Science
In a field that is predominantly preoccupying all of us these days, alerts are also of high relevance and can save lives, just as they can prevent crimes in law enforcement.
Not only since the start of the covid-19 pandemic have scientists around the world advocated for Global Immunological Observatory, which would gather data of clinical surveillance and pathogen sequencing to equip physicians as well as drug researchers with the data they can use for medical decisions and drug design. Building on that, extensive data sets containing global disease test results would support the control of the trajectory of a pandemic.
Just as in law enforcement, physicians, drug designers and political decision makers know which questions to ask, they have the data, but they don’t have the surplus of time for extensive investigation. Alerts can provide them with answers once changes in data are detected.
In the context of a potential Global Immunological Observatory into which health care units around the globe report numbers on cases per disease and medication administered, on individual courses of disease, the amount of data is soon massive. And here lies the opportunity: insights that are relevant for researchers as well as decision makers are buried in the huge amount of data.
For researchers that want to understand the capability of drug repurposing, Hume alerts can help to understand what results the administration of certain drugs had on individual courses of the disease. A pattern could be defined that queries the data for applications of the drug of interest to individual patients. Every time the health status of the patient is updated, an alert can inform the researcher.
For decision makers, Hume alerts can be used to understand the spread of a disease. For example: are certain thresholds reached? In which places have they been broken? An alert would offload decision makers from manually combing the data and proactively checking each location for indicators of interest (such as numbers of new cases).
Conclusion
With graph technologies being more and more widely used, data from heterogeneous sources is available for analysis by highly skilled people such as data analysts, investigators, researchers and political decision makers. Thanks to a powerful ecosystem of sources and a permanent ingestion of data, the amount of data is growing constantly. Yet, the time available for analysis is limited. Hume Alerts offer a shortcut to the data of interest. They offload analysts from conducting exploration steps to uncover the relevant information and enable them to spend more time exploring relevancies. Alerts also ensure that no relevant change in a dataset goes unnoticed.

Alerts in Hume offer great flexibility in formulating the queries that are used by an alert. With Cypher, any question can be formulated and run against the database. Analysts then receive an answer to their questions directly in the graph and can start the exploration of the data without searching for the correct starting point.
For the analog places, where we spend our weekends, snow might work well at some points during the year. But inspired by Hume Alerts, I am now scouting for a monitoring system that can keep the compost bag safe during the summer as well. Suggestions welcome!