In the ever-evolving landscape of financial crime, Anti-Money Laundering (AML) and Know Your Customer (KYC) procedures are critical tools for detecting suspicious activity. As criminals become more sophisticated, so too must the methods used to AML investigations and uncover illicit financial networks. One high-profile case that illustrates the complexity of modern financial crime is the Azerbaijani Laundromat, a large-scale money-laundering operation that funnelled billions of dollars through a network of shell companies.
In the a recently published webinar, our experts demonstrate how connected data analysis tools, can transform the fight against financial crime, enabling institutions to shift from reactive AML investigations to proactive monitoring and real-time risk detection. This article delves into key takeaways from that session, showcasing how connected data analysis and integration techniques can make AML and KYC processes more efficient.
Table of contents:
- The Power of Data Integration in AML Investigations
- Visualising Financial Networks
- Identifying Red Flags Through Data Analysis
- No Ownership Data
- Mailbox Office Addresses
- Suspicious Officers
- Politically Exposed Persons (PEPs)
- Advanced Queries for Analysts
- Time-Scale Visualization and SAR Filing
- Conclusion
The Power of Data Integration in AML Investigations
One of the most powerful aspects of the innovative approach to data analysis using graph technology is its ability to pull data from multiple sources and resolve entities across different datasets. In this case, different disconnected data from the OCCRP, Companies House, and open sanctions lists were combined using GraphAware Hume to provide a fuller picture of LCML Alliance’s activities. For example, the same company appeared in both the OCCRP dataset and the UK Companies House records, but with different pieces of information attached.
Graph technology excels at linking these disparate data points, creating a unified view of the company’s ownership structure, officers, and transactions. The result was a much clearer understanding of how a company operates within the broader network of financial crime.
This ability to integrate data from multiple sources is crucial in modern AML investigations, where criminals often use a combination of fake companies, shell corporations, and cross-border transactions to obscure their activities.
By combining data sources and resolving entities, analysts can identify real-world connections that are deliberately obfuscated by proxy or non-existent shareholders and would otherwise go unnoticed.
Visualising Financial Networks
One of the key challenges in financial crime and AML investigations is understanding the intricate web of transactions, entities, and individuals involved. Traditional approaches often focus on transaction monitoring alone, but this can miss the bigger picture. Connected data analytics platforms like GraphAware Hume, however, offer a powerful alternative by visualising financial networks in a natural way, as a human brain would understand it.
In the Azerbaijani Laundromat case, the Hume platform mapped relationships between bank accounts, organisations, and individuals. The data included information from the Organized Crime and Corruption Reporting Project (OCCRP), detailing transactions between entities such as LCML Alliance LLP and numerous others. By placing this data into a graph format, investigators could easily visualise clusters identifying the most influential companies in the network that funnelled money through the same accounts or shared other suspicious connections.
The graph representation highlighted how certain entities were intertwined in a broader financial network, revealing links that would have been hard to detect through spreadsheets or isolated transactional reports.
Identifying Red Flags Through Data Analysis
Connected data analysis not only improves visualisation but also simplifies the identification of red flags—warning signs that a transaction or entity might be involved in illicit activities. In the webinar, four significant red flags were identified during the Azerbaijani Laundromat investigations:
1. No Ownership Data:
The company LCML Alliance, registered in the UK, lacked any indication of who its ultimate beneficial owner was. There were only companies registered in Seychelles. This absence of transparency is a key indicator of potential fraud.
2. Mailbox Office Addresses:
The AML investigations revealed that the company’s registered address was nothing more than a mailbox, raising further suspicions. This tactic is often used to create the illusion of a legitimate operation while masking the true activities of the business.
3. Suspicious Officers:
The individuals listed as officers of the company were linked to offshore jurisdictions with a history of enabling financial secrecy. This added another layer of suspicion.
4. Politically Exposed Persons (PEPs):
The analysis also identified that some of the transactions eventually funnelled money into the hands of politically exposed persons, further suggesting that the network was being used to launder money.
By integrating data from public sources such as Companies House and open sanctions databases, the GraphAware Hume platform was able to enrich the analysis, connecting LCML Alliance to suspicious individuals and transactions.
Advanced Queries for Analysts
One of the major advantages of using a connected data analysis tool like GraphAware Hume is its ability to make complex queries accessible to non-technical users. Investigators don’t need to be experts in data science to uncover hidden relationships; they can use pre-configured business logic and right-click menus to explore connections within the data.
In the Azerbaijani Laundromat case, analysts were able to trace transactions from LCML Alliance to individual bank accounts with a few clicks. Instead of manually sifting through hundreds of transactions, the tool allowed them to focus on the most relevant relationships quickly and efficiently.
The advanced querying feature also allowed analysts to expand their AML investigations beyond the immediate network, tracing connections to other datasets, such as sanctions lists or politically exposed persons databases. This capability significantly reduces the time required to uncover critical information.
Time-Scale Visualization and SAR Filing
Traversing transactional with geo and time data also opens new opportunities for more efficient risk detection/red flags spotting. GraphAware Hume platform is its time-scale visualisation tool, which allows investigators to track how a network of transactions grows and evolves over time and space. This is particularly useful when monitoring high-risk entities, as it enables investigators to see patterns of activity that might otherwise go undetected.
For example, if an organisation like LCML Alliance has been flagged as suspicious, investigators can use the time-scale tool to track its transaction history. Over time, they can see the scale and speed of how the company’s network expands, providing further evidence that it might be involved in money laundering.
Such a tool is invaluable when deciding whether to file a Suspicious Activity Report (SAR). If a company shows multiple red flags—such as lack of ownership data, mailbox addresses, and connections to PEPs—analysts can file a SAR and flag the entity as high risk. This proactive approach helps prevent the further movement of illicit funds through the financial system.
Conclusion
According to UN statistics, approximately 2-5% of global GDP is laundered money.
The Azerbaijani Laundromat case is older, and just the tip of the iceberg – underscoring the importance of advanced tools in seriously combating financial crime. Traditional transaction monitoring methods, while still valuable, are no longer sufficient to uncover the complex, multi-layered networks that criminals use to launder money. Connected data analysis platforms like GraphAware Hume offer a new way forward, providing investigators with the ability to integrate data from multiple sources, visualise financial networks, identify red flags, and set alerts on suspicious patterns.
By leveraging these tools, financial institutions can strengthen their AML and KYC processes, shifting from reactive AML investigations to proactive monitoring and detection of risk transactions. In a world where financial crime is constantly evolving, staying ahead requires not just vigilance, but also the right technology.
Connected Money: Detecting High-Risk Transactions with Network Analytics
Join our webinar with Senzing and OpenSanctions to discover how combining entity resolution with connected data analytics can tackle the challenges of disparate data sources in tracing financial transactions.
Watch Webinar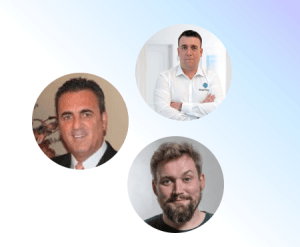