Tax fraud poses a significant challenge for governments and organisations worldwide, resulting in revenue loss, economic instability, and erosion of public trust. Traditional methods of combating tax fraud often fall short due to fraudulent schemes’ complex and constantly evolving nature. These schemes often involve complex networks of entities, transactions, and false documentation, which Relational databases struggle to handle.
However, the advent of knowledge graphs has provided a powerful tool to tackle this pervasive issue head-on. Knowledge Graph (KG) is a structured representation of knowledge that captures entities, their attributes, and relationships. They enable the organisation and retrieval of information in a graph-based format. By leveraging the capabilities of KGs, governments and tax authorities can enhance their fraud detection and prevention strategies, leading to more effective tax compliance and increased revenue collection.
Tax fraud encompasses various illicit activities, and several notable use cases exemplify the challenges tax authorities face.
- VAT return fraud involves manipulating transactions and submitting false invoices to fraudulently claim VAT refunds from the government.
- Carousel fraud, also known as missing trader intra-community (MTIC) fraud, exploits the VAT system by repeatedly buying and selling goods across international borders to generate fraudulent VAT reclaims.
- Phoenix fraud occurs when individuals intentionally liquidate a company to evade tax liabilities and then re-emerge with a new entity to continue business operations.
- Finally, the concept of **Ultimate Beneficial Ownership (UBO) **refers to the identification of real individuals who have control and ownership of an entity, aiming to prevent money laundering, tax evasion, and other illicit activities.
Each of these tax fraud use cases presents unique challenges, but Knowledge Graphs, Neo4j Graph Native database alongside its Graph Data Science library, offers powerful solutions to uncover intricate networks, detect anomalies, and enhance the overall effectiveness of tax fraud detection and prevention strategies.
For this Blogspot, we focus on VAT return fraud, and you can see a video of such a demo below. The features and benefits Hume described, of course, apply to any and all types of Tax fraud.
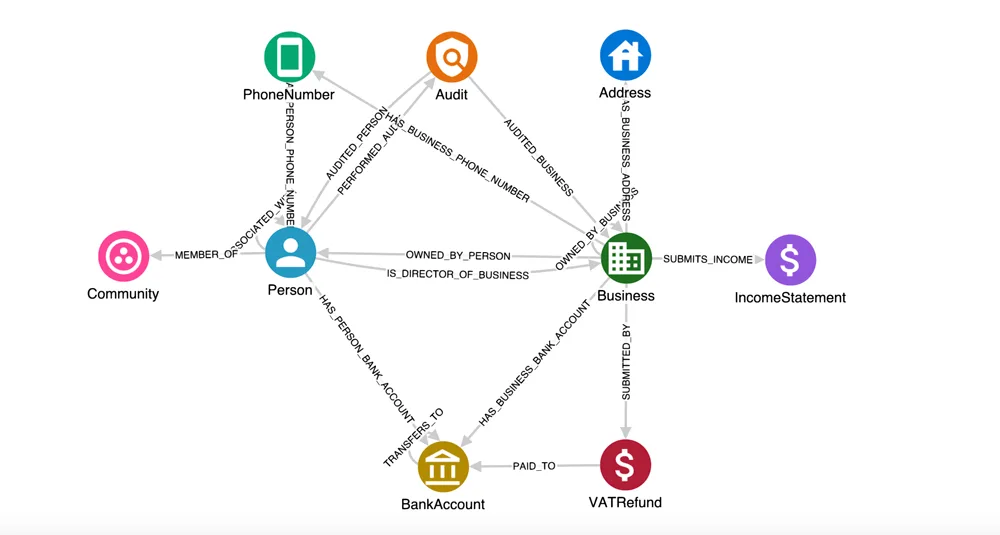
The Role of GraphAware Hume in Countering VAT Return Fraud:
Data Integration
Tax fraud usually involves multiple entities, such as individuals, businesses, and financial transactions. In turn, VAT return fraud investigations require manipulating transactional data, managing unstructured reports, or resolving multiple entities. Hume enables tax authorities to integrate diverse and siloed data sources and then connect all of those to a** single view of intelligence**. It achieves that through Hume Orchestra, its data integration tool that streams data in real-time from a multitude of data sources and enables you to transform them and ingest them all in your Neo4j Database. By cross-referencing this information within a knowledge graph, tax authorities can identify inconsistencies, anomalies, and suspicious patterns indicative of VAT fraud.
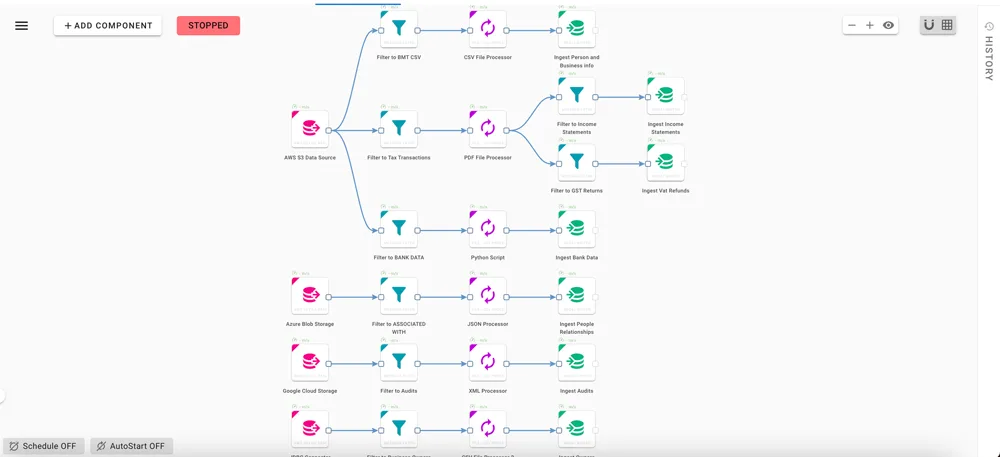
Network Analysis
Knowledge graphs excel at uncovering connections between entities, a crucial aspect in combating VAT return fraud. Tax authorities can construct comprehensive networks that unveil complex fraudulent schemes by linking businesses, owners, bank accounts, and VAT requests. Hume enables your analysts to easily navigate and explore complex relationships on your data through its no-code Features, Actions and Advanced Expand. These help in the identification of hidden patterns, shell companies or money trails, enabling tax authorities to dismantle fraudulent networks effectively.
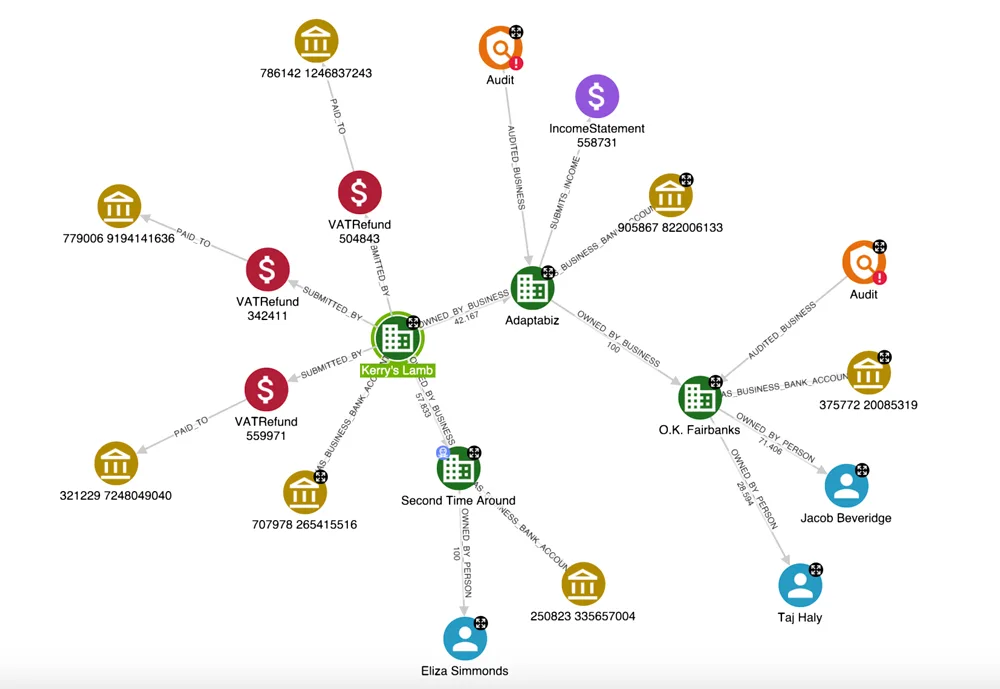
Temporal/Geospatial Analysis
Hume enables the combination of KGs with temporal and geospatial analysis to provide a comprehensive approach to countering VAT return fraud. By incorporating historical VAT data, tax authorities can detect patterns and anomalies over time, while geospatial analysis enables the identification of potential clusters of nodes in close proximity. For example, we could easily focus our investigation on a specific period of time and a specific area which is of interest and leverage the correlation of all the available info to come to executive decisions. This integrated approach enhances fraud detection capabilities, uncovers complex networks, and enables targeted enforcement strategies to mitigate VAT return fraud effectively.
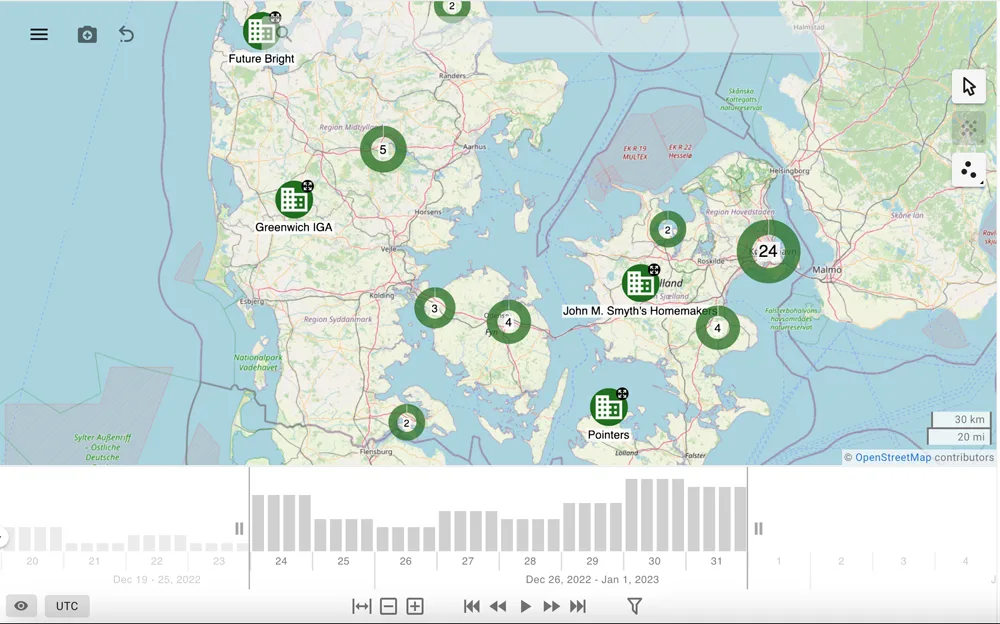
Real-Time Monitoring and Collaboration
Hume Alerts facilitate real-time monitoring of VAT-related activities. By ingesting and processing data in real-time, tax authorities can promptly identify suspicious transactions, inflated claims, or sudden changes in business activities. Automated alerts can notify tax authorities when predefined fraud indicators are detected, enabling swift intervention and preventive measures. Additionally, Hume facilitates collaboration among analysts by providing a unified platform where insights, findings, and investigative leads can be shared and collectively analysed, increasing team productivity.
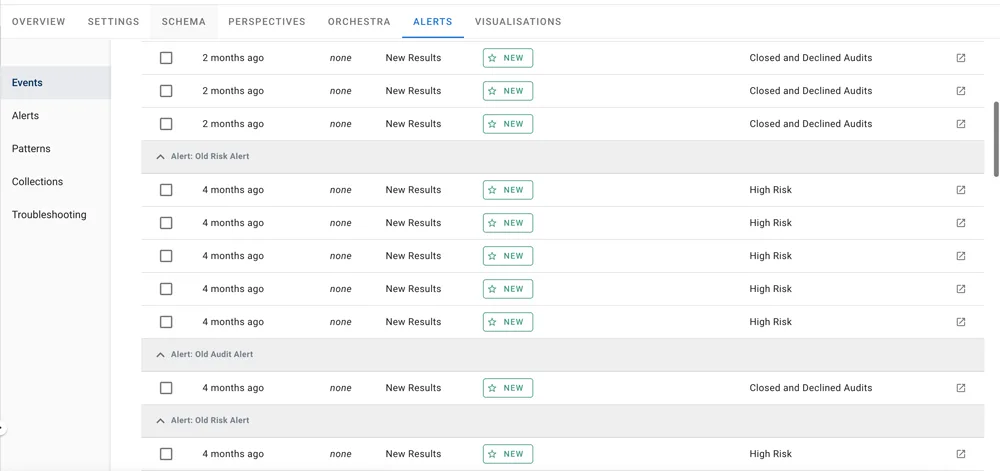
Graph Data Science
In addition to knowledge graphs, Graph Data Science (GDS) techniques play a crucial role in countering VAT return fraud. We can leverage Neo4j’s GDS library, which offers a range of advanced analytical methods designed explicitly for graph data, providing valuable insights for tax fraud detection and prevention. Community detection algorithms enable analysts to identify clusters or groups of entities that exhibit similar patterns of fraudulent behaviour. By detecting these communities, tax authorities can better understand the structure of fraudulent networks and target their investigations more effectively. Network centrality measures, such as degree centrality and betweenness centrality, help identify key entities within a fraud network that play critical roles in facilitating fraudulent activities. These centrality measures highlight influential individuals or entities that may be orchestrating or benefiting from tax fraud schemes. Furthermore, graph embeddings can capture the latent features of entities and their relationships in a network. This enables analysts to compare entities, detect anomalies, and predict potential fraud risks based on the learned representations.
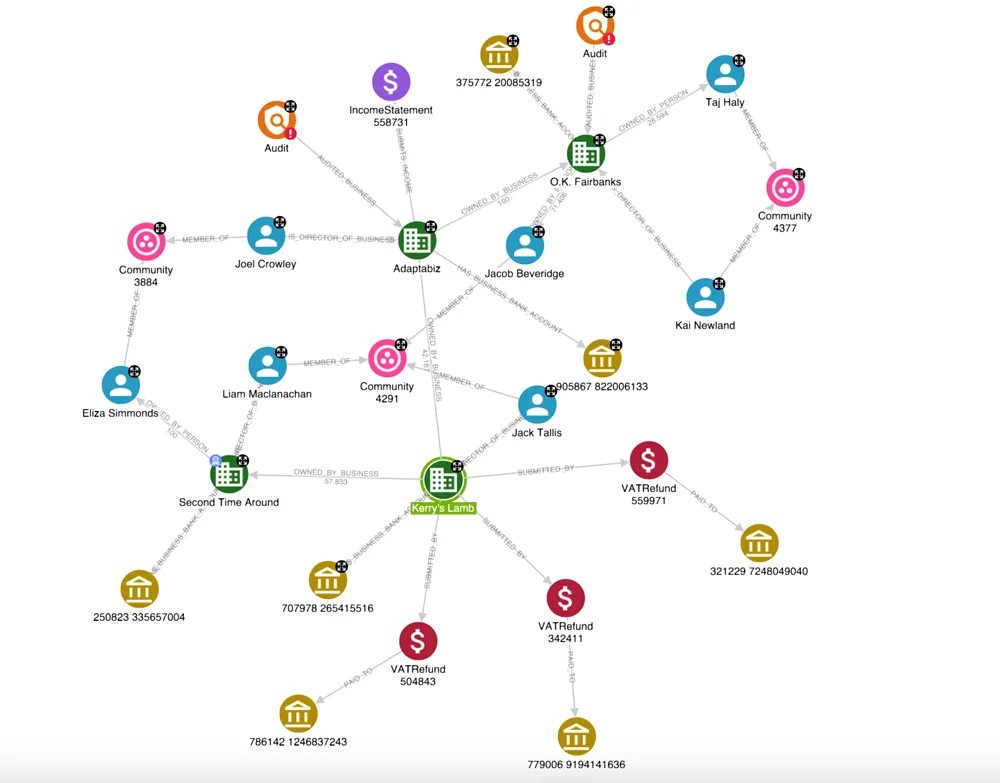
Conclusion
Tax fraud represents a critical challenge for tax authorities, requiring innovative approaches to counter it efficiently. Having your data in Hume offers a powerful solution by leveraging the power of Knowledge graphs, Neo4j Graph Native database and by integrating data, establishing connections between entities, enabling advanced analytics and alerting in real-time the tax authorities. This way, tax authorities can enhance their detection capabilities, conduct comprehensive network analysis, predict fraudulent behaviours and take precautionary measures to save millions of Euros/Dollars per month.