Fusion analysis is commonly understood as the process of integrating data from multiple sources to gain a more comprehensive understanding of complex systems. In this article, we’ll delve into the intricacies of fusion analysis, exploring its definition, mechanisms, benefits, applications, and its significance in various fields, especially intelligence and law enforcement.
What is Fusion Analysis?
Fusion analysis is like putting together a giant puzzle. Instead of picture pieces, you have data points from different sources – think financial transactions, social media posts, or intelligence reports. By connecting these dots, you can see the bigger picture, uncover hidden patterns, and make informed decisions.
Visualizing this data in a graph format, where nodes represent entities (people, organizations, events) and edges represent relationships between them, can make complex information more understandable. This visual representation helps analysts quickly identify trends, anomalies, and potential threats.
How Does Fusion Analysis Work?
Fusion analysis involves several key steps:
- Data Acquisition: Gathering data from various sources, such as databases, social media, and intelligence reports.
- Data Cleaning and Preprocessing: Cleaning and transforming the data to ensure consistency and accuracy.
- Data Integration: Combining data from different sources into a unified format, often represented as a graph.
- Graph Analysis: Applying graph algorithms to analyze the relationships between entities and identify patterns, anomalies, and communities.
- Visualization: Creating visual representations of the analyzed data to facilitate understanding and decision-making.
By following these steps, analysts can uncover hidden connections, predict future trends, and make data-driven decisions.
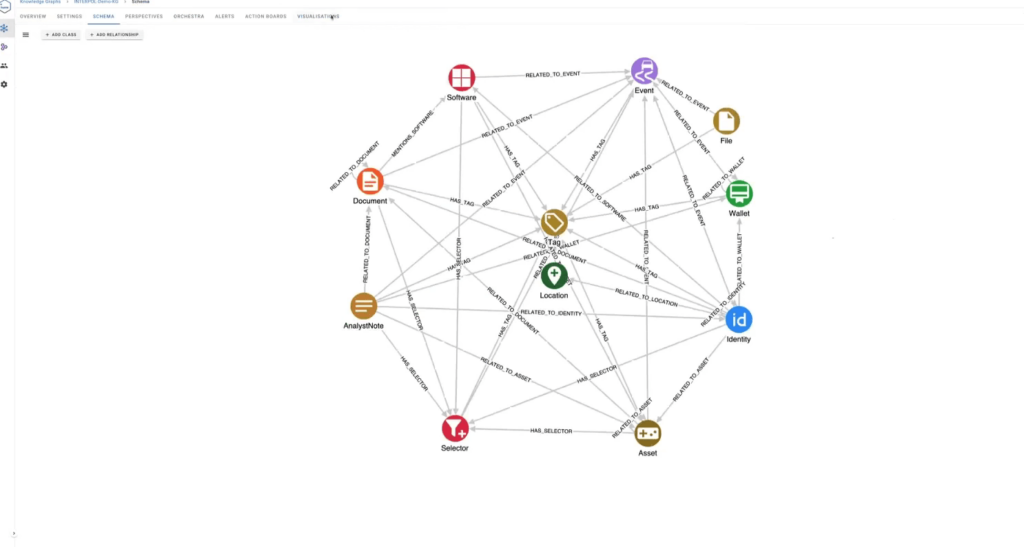
What Are the Benefits and Advantages of Fusion Analysis?
Fusion analysis offers numerous benefits:
Faster Insights
By automating data integration and analysis processes, fusion analysis can significantly speed up the time it takes to derive insights from large and complex datasets.
Improved Accuracy
By combining multiple data sources, fusion analysis can reduce the risk of errors and biases that may arise from relying on a single source of information.
Deeper Insights
Fusion analysis can uncover hidden relationships and patterns that may not be apparent when analyzing data in isolation. By connecting the dots between different data points, analysts can gain a more comprehensive understanding of complex systems.
Enhanced Decision-Making
By providing timely and accurate insights, fusion analysis can support better decision-making in a variety of fields, from intelligence and law enforcement to healthcare and finance.
What Are the Applications of Fusion Analysis?
Fusion analysis has a wide range of applications across various industries:
Financial Fraud Detection
By analyzing financial transactions, social media data, and other relevant information, fusion analysis can help identify suspicious activities, such as money laundering and fraud.
Criminal Intelligence
Law enforcement agencies can use fusion analysis to connect the dots between different crimes, identify criminal networks, and predict future criminal activity.
National Security
Intelligence agencies can leverage fusion analysis to track terrorist activities, monitor cyber threats, and assess geopolitical risks.
Healthcare
Healthcare providers can use fusion analysis to analyze patient records, genomic data, and clinical trial data to improve patient outcomes and develop new treatments.
Cybersecurity
Cybersecurity analysts can use fusion analysis to detect and respond to cyberattacks by analyzing network traffic, log data, and threat intelligence.
What is the Difference Between Fusion, Predictive, and Network Analysis?
While these terms are often used interchangeably, they have distinct meanings:
- Fusion Analysis: Combines data from multiple sources to gain a comprehensive understanding of a system.
- Predictive Analysis: Uses statistical models and machine learning algorithms to forecast future trends and outcomes.
- Network Analysis: Focuses on the relationships between entities, such as people or organizations, within a network.
In many cases, fusion analysis involves elements of both predictive and network analysis. By combining data from various sources and analyzing the relationships between entities, analysts can make more accurate predictions and identify potential threats.
What Are Fusion Analysis Software and Tools?
Several software tools and platforms are designed to facilitate fusion analysis. These tools often incorporate graph databases, machine learning algorithms, and visualization techniques to help analysts extract valuable insights from complex datasets.
While many general-purpose data analysis tools can be used for fusion analysis, specialized graph database solutions like Neo4j and Amazon Neptune are particularly well-suited for this task. These tools enable efficient storage, querying, and analysis of complex relationships between entities.
GraphAware offers a suite of tools and libraries that can be used to build and analyze knowledge graphs, which are a powerful tool for fusion analysis. These tools can be used to extract knowledge from unstructured data, build knowledge graphs, and perform advanced analytics on these graphs.
By leveraging these tools, analysts can streamline the fusion analysis process and uncover deeper insights.
What Does Fusion Analysis Mean Within Big Data?
In the era of big data, where vast amounts of information are generated daily, fusion analysis becomes even more critical. It enables organizations to extract meaningful insights from this data deluge by combining data from diverse sources and analyzing it holistically.
By applying fusion analysis techniques to big data, organizations can:
- Identify complex patterns and relationships: Uncover hidden connections between seemingly unrelated data points.
- Make more accurate predictions: Develop more precise forecasting models by incorporating multiple data sources.
- Improve decision-making: Gain a deeper understanding of the factors influencing business outcomes.
- Enhance operational efficiency: Optimize processes and reduce costs by identifying inefficiencies.
As the volume, velocity, and variety of data continue to grow, fusion analysis will play an increasingly important role in helping organizations harness the power of big data.
Why is Fusion Analysis Important in Law Enforcement?
Fusion analysis has revolutionized the way law enforcement agencies operate. By integrating data from various sources, such as criminal databases, social media, and intelligence reports, law enforcement can:
- Identify emerging threats: Detect potential threats early on by identifying patterns and anomalies in data.
- Prioritize investigations: Focus resources on high-priority cases by assessing the risk level of different threats.
- Improve investigative efficiency: Accelerate investigations by quickly identifying leads and connecting the dots between different pieces of evidence.
- Enhance public safety: Protect citizens by preventing and responding to crime more effectively.
How Does Fusion Analysis Help Intelligence Agencies and Police?
Here are some specific use cases of fusion analysis in law enforcement:
- Predictive Policing: By analyzing historical crime data, law enforcement agencies can identify areas at risk of future crime and deploy resources proactively.
- Terrorism Investigation: Fusion analysis can help identify terrorist networks, track their activities, and disrupt their plans.
- Organized Crime: By analyzing financial transactions, social media data, and other information, law enforcement can dismantle criminal organizations and seize their assets.
- Human Trafficking: Fusion analysis can help identify and rescue victims of human trafficking by analyzing travel patterns, financial transactions, and social media data.
By leveraging the power of fusion analysis, law enforcement agencies can make data-driven decisions that can save lives and protect communities.
What Are Examples of Fusion Analysis Use Cases?
Fusion analysis has a wide range of applications across various industries. Here are a few examples:
Business Analytics
- Customer Segmentation: By analyzing customer data from multiple sources, businesses can identify distinct customer segments and tailor marketing campaigns accordingly.
- Fraud Detection: Fusion analysis can help detect fraudulent activities, such as insurance fraud or credit card fraud, by identifying unusual patterns in data.
- Supply Chain Optimization: By analyzing supply chain data, businesses can optimize inventory levels, improve delivery times, and reduce costs.
Healthcare
- Disease Outbreak Detection: By analyzing patient records, social media data, and other relevant information, healthcare organizations can quickly identify and respond to disease outbreaks.
- Personalized Medicine: Fusion analysis can help identify genetic markers and other factors that influence patient response to treatment, leading to more personalized and effective therapies.
Criminal Investigation
- Link Analysis: By analyzing relationships between people, organizations, and events, law enforcement can identify criminal networks and uncover hidden connections between crimes.
- Geographic Profiling: Fusion analysis can help predict the location of future crimes by analyzing historical crime data and other relevant factors.
By leveraging the power of fusion analysis, organizations can make data-driven decisions that can improve efficiency, reduce costs, and enhance overall performance.