Over 10,000 physical typewritten documents from 1932 to 1941 had to be digitised, structured, and connected in order to create a single, centralised source of knowledge, for enabling the analysis of historical processes. The ensuing process of going from natural text to knowledge graph, was both complex yet rewarding.
The Rockefeller Archive Center (RAC) is a major repository andresearch centre for the study of philanthropy and its impact throughout the world. The specific research project revolves around the process of grantmaking, the distribution of funding, the formation of intellectual networks and the transfer and adoption of knowledge.
The RAC wanted the capability to swiftly obtain answers to inquiries such as: “Are there any patterns that typically precede the funding of an idea?” and “Do granted projects tend to run through recommendations of influential scientists or previous grantees?”. In order to achieve this, the historical records were brought to life via complex machine learning pipelines, to extract the wealth of knowledge within and modelling it as a knowledge graph.
The project was launched to create a model that could demonstrate how structured data created from analogue, textual records can support a broader range of historical researchers working in quantitative methodologies. With many of the organisations whose records are held by the archive transitioning to paperless and digital platforms, the project was initiated to enhance ways of using historical records.
The Rockefeller Archive Center selected GraphAware as their vendor of choice due to the dynamic capabilities of the GraphAware Hume knowledge graph platform and the experience of GraphAware data scientists with unstructured data.
The Challenges – 10,000 Unstructured Documents
As previously mentioned, the project had a set of clearly defined objectives:
- Demonstrate the potential of digitising analogue historical records of various types and combining and connecting them within a single searchable graph database.
- Design advanced Machine Learning pipelines to extract knowledge from the documents, text to knowledge graph.
- Identify new insights and patterns of grantmaking by examining intellectual networks and the development of research fields over time.
- Provide advanced analytics tools to allow researchers from a broad range of quantitative disciplines to engage with the records held by the Rockefeller Archive Center.
The primary challenge in this project was to extract structured information from the unstructured content of physical typewritten historical documents. To accomplish this task, a system that integrates graph-based technologies and Natural Language Processing (NLP) had to be designed and implemented. The stages of this process included:
- Transforming scanned documents into a clean textual content.
- Extracting the relevant relational knowledge locked within the texts.
- Representing the extracted knowledge in a rich graph structure.
- Providing an interface to query, analyse and visualise the highly complex graph, allowing for a comprehensive understanding of the connections between the documents and facilitating new discovery opportunities.
The project focused on two types of records, consisting of a total of approximately 12,000 pages dating from 1932 to 1941.
- The Rockefeller Foundation Officer Diaries are detailed textual records of meetings, calls and various discussions among Rockefeller program officers and scientists, universities and other influencers about ongoing and planned research seeking funding.
- The Rockefeller Foundation Board of Directors’ Minute Books provide information on the grants that were awarded by the Board, such as the receiving university or organisation, the research topic, and the amount.
The historical nature of the original data, the domain-specific linguistic patterns and the complexity of the relational knowledge contained within all suggest that extracting and modelling all relevant information from these physical documents is highly challenging.
The Solution – From Text to Knowledge Graph
To address the challenge, the Data Science Team at GraphAware designed a schema and deployed a solution built on the following pillars:
- Optical Character Recognition document parsing (OCR)
- Customised document chunking to extract diary entries
- Named Entity Recognition
- Entity Relation Extraction
- Entity Resolution to link different data records referring to the same entity
- Graph algorithms/analytics to analyse the intellectual network (identify influencers etc.)
- Analyse time evolution of research disciplines
- Configure Hume Actions to allow users to answer relevant questions as specified by RAC
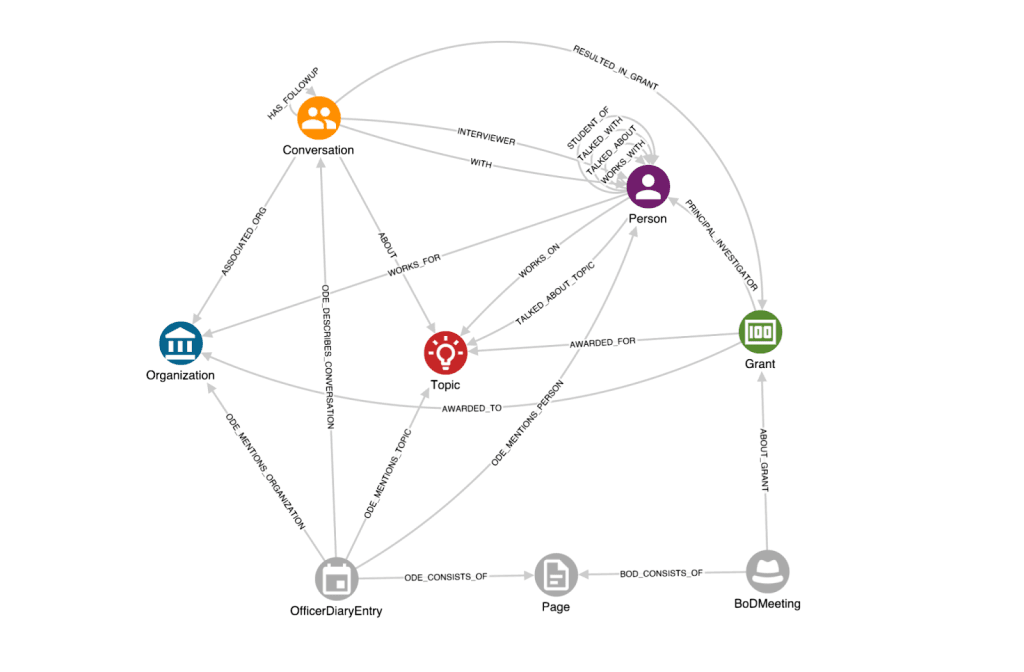
The Rockefeller Archive Center and GraphAware leveraged the power of Large Language Models (LLMs) to address a variety of complex tasks. These efforts include document chunking to identify diary entries, Named Entity Recognition and Relation Extraction, as well as Entity Resolution through the normalisation of well-known organisation names. A key aspect of this work involved the strategic use of multiple language models to effectively handle tasks of varying complexity and ensure accuracy.
Are you curious to discover how advanced analytics & LLMs can be utilised to effectively extract knowledge from unstructured data?
The Results – See Patterns That Led to a Grant Being Approved
The technical solution, which included OCR parsing, complex customised NLP processing and graph data scienceto connect and mine the texts from thousands of pages and model them within a knowledge graph, was completed and ready for use within six months. This enabled analysts to effectively answer important questions through the use of graph visualisation, customised by Hume Actions.
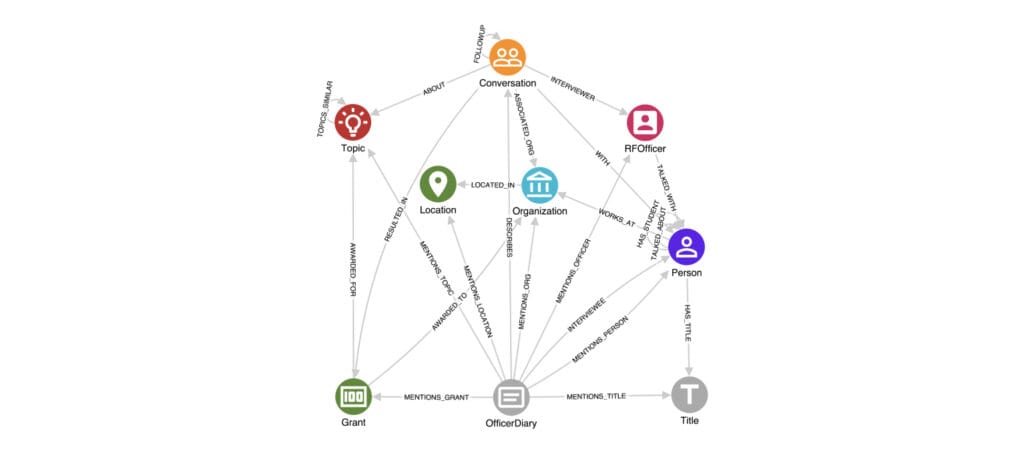
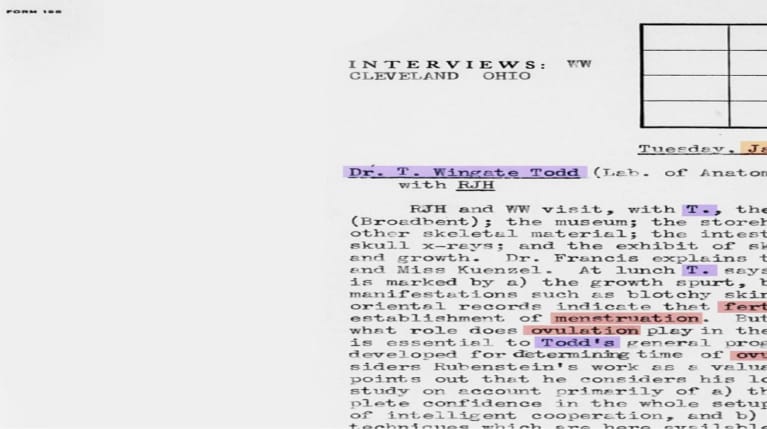
The Actions feature, which includes predefined Cypher queries, allows analysts to easily and quickly retrieve answers to specific questions such as the number of conversations required to secure a grant, key influencers in the grant-making process, and the impact of preexisting connections within the organisation.
According to the RAC, Hume is a highly advanced and powerful insights and analytics engine; they had not seen anything similar in archival conferences addressing data management. The knowledge graph not only provides insight into past events, such as patterns that led to a grant being approved but also enables users to trace research roads not taken and pathways which did not result in funding.
We are delighted to say that the RAC team stated that, in retrospect, the success of the project would have not been possible without the support and guidance of GraphAware’s Data Science Team.
The Future
The success of digitising analogue historical records and creating a queryable knowledge graph using GraphAware Hume, demonstrated the potential and power of cutting-edge text to knowledge graph technology.
GraphAware is not just a vendor, we gained an intellectual partner
— Director of Research & Education RAC