Intelligence analysis is the process of examining information and data to understand its significance, draw conclusions, and make predictions.
What is Intelligence Analysis?
In its essence, intelligence analysis bridges the gap between raw information and actionable knowledge. It aims to provide insights and assessments that inform decision-making.
Intelligence analysis can cover a wide range of topics, from national security and policy making to business, and even personal issues. Successful intelligence analysis must involve critical thinking, research, data analysis, and may use specialised tools and techniques.
Its outputs are usually reports, briefings, policy notes and other forms of communication that convey key findings and recommendations that are not based on personal opinions or biases.
How does Intelligence analysis work?
For the best results, intelligence analysis has to be systematic in gathering and examining relevant information and data to understand its significance, draw conclusions, and make relevant, useful predictions.
The data for intelligence analysis is usually collected from diverse sources in order to provide the most accurate picture. They can be split into several categories:
Open-source intelligence (OSINT): Publicly available information like news articles, social media, and government documents.
Human intelligence (HUMINT): Information gathered from human sources, such as spies, informants, and defectors.
Signals intelligence (SIGINT): Intercepted communications, such as phone calls, emails, and radio transmissions.
Imagery intelligence (IMINT): Information gathered from satellites, drones, and other aerial platforms.
Measurement and signature intelligence (MASINT): Technical intelligence gathered from various sources, such as radar and seismic sensors. Social media intelligence (SOCMINT): Information gathered from social media is becoming increasingly more relevant for intelligence analysis.
Key Objectives of Intelligence Analysis
The main objectives of intelligence analysis are:
Providing timely and accurate assessments – analyzing information to understand current situations, identify emerging threats, and predict future events.
Informing decision-making – providing actionable intelligence that helps decision-makers understand the implications of different courses of action and make informed choices.
Preventing or mitigating threats – by identifying and understanding threats, intelligence analysis can help to prevent them from occurring or mitigate their impact.
Protecting national security – intelligence analysis plays a critical role in protecting a country from external threats and internal vulnerabilities.
Supporting law enforcement – by providing relevant information, intelligence analysis can help law enforcement agencies to investigate crimes, apprehend criminals, and disrupt criminal activities.
Gaining competitive advantage in business – intelligence analysis can help companies to understand their competitors, identify market trends, and make informed business decisions.
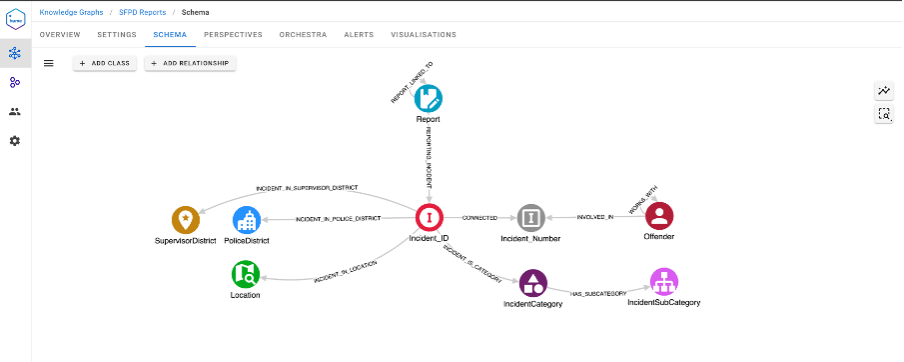
Core Techniques in Intelligence Analysis
Intelligence analysis uses a combination of qualitative and quantitative techniques to derive as much useful information from the existing intelligence as possible. They can be split into several categories:
- Structured Analytic Techniques (SATs) provide a framework for analysts to think critically, challenge assumptions, and produce more robust and reliable assessment. Some of the wider used SATs are:
- Delphi Method: A structured process for eliciting expert opinions on a particular topic.
- Red Teaming: Involves challenging assumptions and conclusions by simulating an adversary’s perspective.
- Event Tree Analysis: A graphical technique used to identify potential consequences of a particular event.
- Influence Diagrams: A visual representation of the relationships between different variables in a decision-making problem.
- Analysis of Competing Hypotheses (ACH): A systematic approach to evaluating multiple hypotheses by systematically testing them against available evidence.
- SWOT Analysis: A structured approach listing main strengths, weaknesses, opportunities and threats of an approach, scenario or a competitor. It’s best used in combination with other SATs so as not to oversimplify a complex situation.
- Data analysis and visualisation are used to recognise patterns in the data, explore and discover new relationships in existing data and discover anomalies. They are also highly important when communicating the results and recommendations to policymakers and other stakeholders.
Among the most common techniques are:- Graphs: Visualise relationships between entities (people, organisations, locations) in networks. This helps identify clusters, key players, and information flows within complex networks.
- Heatmaps: Highlight areas of high activity or concentration, revealing patterns and anomalies in data sets. For example, a heatmap could show areas with high volumes of communication or suspicious activity.
- Visualisations: Allow analysts to quickly explore large datasets and identify trends, outliers, and unexpected relationships that might be missed in raw data.
- Interactive Dashboards: Enable analysts to dynamically filter, sort, and explore data, gaining deeper insights and uncovering hidden connections.
- Outlier Detection: Visualisations can help identify unusual patterns or activities that may indicate threats or suspicious behavior. For example, a sudden spike in communication between two individuals or a cluster of unusual activity in a specific location.
- Link Analysis: Visualising social networks, communication patterns, and financial transactions to identify relationships between individuals and organisations.
- Geolocation Data: Using heatmaps to visualise the movement of individuals or the distribution of resources in geographical space.
- Timeline Analysis: Visualizing events over time to identify trends, patterns, and potential causal relationships.
What are the benefits and advantages of intelligence analysis?
Intelligence analysis offers several key advantages for decision makers and other stakeholders. They are most notably speed, efficiency and relevance for the analyzed scenario. They can be summarised as:
- Improved Decision-Making: Reduces uncertainty, enhances risk assessment, and informs strategic planning.
- Increased Awareness: Provides early warnings of threats and improves crisis response.
- Enhanced Security: Supports counterterrorism, cybersecurity, and national security efforts.
- Competitive Advantage: Provides valuable insights for businesses in areas like market research and competitor analysis.
- Efficiency and Productivity: Improves resource allocation and supports data-driven decision-making.
- Early Warning of Threats: By monitoring information sources, intelligence analysis can provide early warnings of potential threats, such as cyberattacks, natural disasters, or geopolitical instability.
- Improved Crisis Response: Enhanced situational awareness enables faster and more effective responses to crises.
What are the types of intelligence analysis?
Intelligence analysis is used differently in different fields, although the main goals are generally the same – to achieve the strategic goals of the organisation more efficiently through the use of wide array of available data.
Business Intelligence (BI) analysis is the process of using data to gain valuable insights into a company’s operations. It involves collecting data from various sources, analyzing it using tools and techniques, and transforming the findings into actionable insights. These insights help businesses make informed decisions, improve efficiency, increase revenue, gain a competitive advantage, and enhance customer satisfaction.
Market intelligence analysis is similar to BI, but focuses on gathering and analyzing data about market trends, customer behavior, competitor activities, and industry dynamics.It Enables businesses to make informed decisions about product development, marketing strategies, pricing, and overall business strategy.
Law Enforcement Intelligence Analysis involves collecting and analyzing information to identify, investigate, and disrupt criminal activity. It utilises techniques like crime mapping, link analysis, and data mining to identify patterns, predict future criminal activity, and apprehend offenders.
Military Intelligence Analysis gathers and analyzes information about potential adversaries, threats, and the operational environment to support military planning and operations. Covers areas such as enemy capabilities, intentions, and vulnerabilities, as well as the impact of terrain, weather, and other environmental factors.
What are the applications of intelligence analysis?
Intelligence analysis has a wide range of applications across various sectors, both public and private. As the volume and complexity of existing and emerging data continue to grow, the importance of intelligence analysis will only increase. The main application areas are:
National Security
- Counterterrorism: Identifying and tracking terrorist activities.
- Counterintelligence: Countering espionage, sabotage, and foreign interference.
- Military Operations: Planning and executing military campaigns, assessing enemy threats.
- WMD Proliferation: Monitoring the development and spread of weapons of mass destruction.
Law Enforcement
- Crime Investigation: Identifying and apprehending criminals, disrupting criminal organisations.
- Counter-narcotics Operations: Combatting drug trafficking and organised crime.
- Cybercrime Investigation: Investigating and combating cyber threats.
Business
- Competitive Intelligence: Understanding competitors, market trends, and customer behavior.
- Market Research: Identifying new market opportunities and developing effective marketing strategies.
- Risk Management: Identifying and mitigating potential risks to the business.
Public Health
- Disease Surveillance: Monitoring the spread of infectious diseases and identifying potential outbreaks.
- Public Health Emergencies: Responding to public health crises, such as pandemics.
Finance
- Fraud Detection: Identifying and preventing financial fraud. In most jurisdictions, the regulation obliges the financial institutions to prevent money laundering or tax evasion and properly identify each individual attempting to make transactions.
- Risk Assessment: Assessing financial risks and developing risk mitigation strategies e.g. for loan provision to persons and businesses.
Academia and Research
- Social Science Research: Analyzing social and political trends.
- Historical Research: Understanding past events and their impact on the present.
- Avoiding Plagiarism and Fraud: Checking the academic papers for signs of plagiarism and potentially fraudulent results that could tamper existing research.
What are Intelligence Analysis Software and Tools?
Intelligence analysis relies on a variety of software tools to effectively process and analyze information. They differ in their complexity and usability, but the most important qualities to check is their security and ability to interact with a wide selection of data sources.
Tools and Technologies Used
Software tools for intelligence analysis fall into several categories.
Data Collection & Management:
OSINT Tools: Tools for collecting and analyzing data from open sources like social media, news articles, and public records (e.g., Maltego, Hootsuite, Google Alerts).
Data Warehouses & Lakes: Platforms for storing and managing large volumes of structured and unstructured data (e.g., Amazon S3, Google Cloud Storage, Snowflake).
Data Analysis & Visualisation:
Business Intelligence Platforms: Tools for data analysis, reporting, and visualisation (e.g., Tableau, Power BI, Qlik Sense, Palantir).
Statistical Software: Tools for statistical analysis and data mining (e.g., R, Python, SPSS).
Network Analysis Software: Tools for visualising and analyzing relationships between entities in networks (e.g., Gephi, NodeXL, i2 and GraphAware Hume).
Geospatial Analysis Software: Tools for analyzing geographic data, such as crime mapping and location intelligence (e.g., ArcGIS, QGIS).
Role of AI and machine learning in modern intelligence analysis
AI plays a transformative role in intelligence analysis software, offering significant advantages in all relevant areas. AI can help process massive datasets at unprecedented speeds, identifying patterns and anomalies that would take human analysts much longer to detect manually.
AI automates repetitive tasks like data entry, information extraction, and report generation, freeing up analysts to focus on higher-level analysis.
AI tools are able to identify complex patterns and relationships within data, revealing hidden or intentionally obfuscated connections between individuals, organisations, and events. They are able to automatically flag unusual activities or deviations from normal behavior, which can indicate potential threats.
With the help of Natural Language Processing the AI is able to analyze text data, such as news articles, social media posts, and intelligence reports, to extract key information and their relationship, identify sentiment, and understand the context.
AI can also help with faster and more informed decision-making in critical situations, such as counterterrorism operations and disaster response.
Depending on the datasets used to train AI, AI can help to mitigate some human biases by providing objective and data-driven insights.
Challenges in Intelligence Analysis
The challenges stem mainly from the rapidly evolving environment that produces both large amounts of data and new technologies. This requires continuous innovation in analytical techniques, the development of robust data management systems, and a strong emphasis on ethical considerations and human oversight.
The main challenges in intelligence analysis are:
Data Overload: The sheer volume of data available from various sources (social media, open-source, intelligence reports) can be overwhelming and difficult to process effectively.
Data Quality: Ensuring the accuracy, reliability, and completeness of data is crucial, but often challenging. Information can be incomplete, inaccurate, or deliberately misleading.
Cognitive Biases: Analysts, like all humans, are susceptible to cognitive biases that can distort their judgment and lead to flawed conclusions. These biases include confirmation bias, anchoring bias, and availability bias.
Technological Challenges: Rapidly evolving technologies, such as artificial intelligence and machine learning, present both opportunities and challenges. Ensuring ethical and responsible use of these technologies while mitigating potential risks is crucial.
Ethical Considerations: Intelligence analysis often involves sensitive information and raises ethical concerns regarding privacy, surveillance, and the potential for misuse of information.
Resource Constraints: Limited budgets, personnel, and technological resources can constrain the ability to effectively collect, analyze, and disseminate intelligence.
Counterintelligence Threats: Adversaries actively work to counter intelligence gathering efforts, making it difficult to obtain accurate and timely information.The Evolving Threat Landscape: The nature of threats is constantly evolving, requiring analysts to adapt their methods and approaches to address new challenges, such as cyber threats and the spread of disinformation.
What is Law Enforcement Intelligence analysis and why is it important?
Law enforcement intelligence analysis is the process of collecting, analyzing, and interpreting information to understand criminal activity, identify threats, and support investigative efforts.
It can be used for:
- Proactive Policing: Moves beyond reactive responses to crime. By analyzing trends, patterns, and connections, law enforcement can anticipate and prevent crime before it occurs.
- Targeted Investigations: Helps focus investigative resources on the most significant threats, such as organised crime, terrorism, and drug trafficking.
- Improved Resource Allocation: Enables more efficient deployment of personnel and resources by identifying crime hotspots and predicting future crime trends.
- Enhanced Situational Awareness: Provides law enforcement agencies with a better understanding of the criminal landscape and the evolving nature of threats.
- Improved Decision-Making: Supports informed decision-making at all levels, from tactical operations to strategic planning.
Key Techniques:
- Crime Mapping: Analyzing the spatial distribution of crime to identify patterns and hotspots.
- Link Analysis: Identifying connections between individuals, organisations, and events within criminal networks.
- Data Mining: Using advanced analytical techniques to uncover hidden patterns and relationships within large datasets.
- Predictive Modeling: Forecasting future crime trends and identifying potential targets for intervention.
By effectively utilising intelligence analysis and tools such as GraphAware Hume, law enforcement agencies can enhance their effectiveness, improve public safety, and better serve their communities.
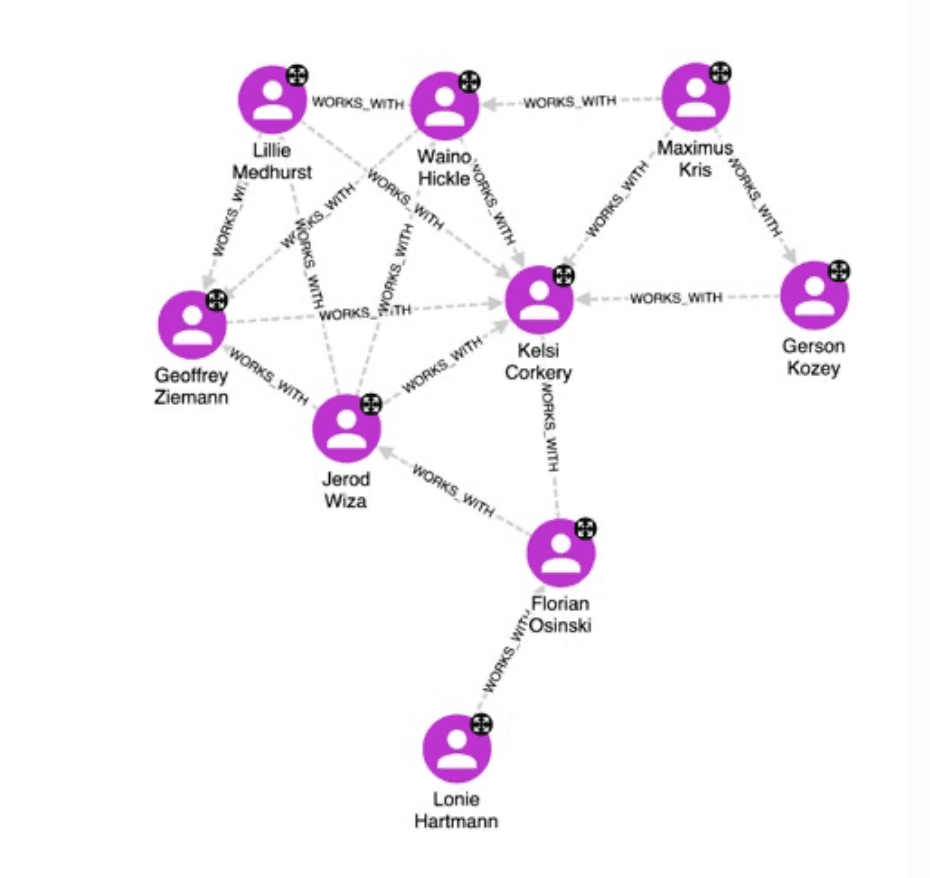
What is the future of Intelligence Analysis?
The future of intelligence analysis will be significantly shaped by several key trends:
- AI and Machine Learning Dominance will play an increasingly critical role in processing vast amounts of data, identifying patterns, and predicting future events. Expect to see advancements in natural language processing (NLP), computer vision, and predictive modeling.
- Focus on Human-Machine Collaboration will be necessary in AI-driven future, leading to a symbiotic relationship between human analysts and AI systems. AI can augment human capabilities by automating routine tasks, identifying anomalies, and providing data-driven insights, while human analysts provide critical judgment, context, and ethical oversight.
- Even more data-driven intelligence is expected in the near future, with a focus on collecting, analyzing, and integrating data from diverse sources. For these, tools able to pool and connect disparate data such as GraphAware Hume are necessary.
What are some GraphAware use case examples in intelligence analysis?
GraphAware has demonstrated the power of graph-based intelligence analysis across various industries, including business analytics, healthcare, and criminal investigation. By leveraging graph technology, organizations can uncover hidden relationships, optimize decision-making, and enhance operational efficiency.
Business Analytics
Business Analytics solutions have been instrumental in enabling businesses to gain deeper insights into their operations and customer relationships. For example, in the case of LPL Financial, GraphAware helped develop a “Single Brain” platform that integrates and analyzes vast amounts of financial data. This platform allows LPL Financial to visualize complex relationships between clients, advisors, and products, enabling better decision-making and personalized service delivery. The graph-based approach has streamlined operations and improved compliance processes. Read more about this case study here.
Criminal Investigation
Hume has played a pivotal role in transforming criminal investigations by enabling law enforcement agencies to analyze complex networks of relationships and activities. A notable example is the work done with Western Australia Police, where the Hume platform was used to enhance intelligence analysis and operational decision-making. The platform allowed investigators to visualize connections between individuals, locations, and events, significantly improving the efficiency and accuracy of criminal investigations. Explore this case study in detail here.