Welcome back to our blog series on unlocking the complex world of ultimate beneficial ownership (UBO) investigations using the power of graph technology. We’ll delve into the various types of investigations that can be conducted with the aid of graph analysis, showcasing the agility, speed, and capability facilitated by tools like GraphAware Hume, our graph analytics platform.
Graph Schema: The Foundation of Investigation
At the heart of GraphAware Hume, lies a robust graph schema – providing a logical representation of entities and their relationships. For our demonstration, we’ve crafted a simple schema encompassing persons, organisations, sanction authorities, and values, offering a structured framework to analyse complex ownership networks.
Data Ingestion and Integration
The effectiveness of UBO investigations heavily relies on the quality and diversity of data sources. Leveraging Open Ownership Statements and subsets of Open Sanctions data, we illustrate the seamless ingestion process into our graph database, Neo4j. This ingestion capability ensures that disparate datasets conforming to standardised formats like BODS (Beneficial Ownership Data Standard) can be efficiently integrated, laying the groundwork for comprehensive analysis.
Investigative Agility: Entity Resolution and Grouping
One of the key challenges in UBO investigations is entity resolution, especially when dealing with variations in entity names and attributes across datasets. With Hume’s entity resolution capabilities powered by Senzing, we effortlessly unify entities, identifying potential duplicates and consolidating them into cohesive entity groups. Moreover, through advanced grouping techniques, we uncover familial relationships within ownership structures, enhancing our understanding of complex networks.
Uncovering Insights: Advanced Querying and Visualization
GraphAware Hume leverages graph technology to empower analysts to navigate complex ownership structures with unparalleled ease. By executing simple queries, such as identifying ownership paths spanning multiple levels or pinpointing entities linked to sanctioned jurisdictions, we demonstrate the agility and depth of analysis afforded by graph-based approaches. Visualising these insights in graph format provides a comprehensive view of interconnected entities, transcending the limitations of traditional tabular representations.
Automation and Monitoring for Proactive Insights
Lastly, we will highlight the importance of proactive monitoring in UBO investigations. Through GraphAware Hume’s monitoring capabilities, analysts can define patterns of interest, such as identifying newly sanctioned entities or detecting secretive ownership structures. By automating these detection processes and receiving real-time alerts, analysts can stay ahead of emerging risks and swiftly respond to evolving scenarios, ensuring robust compliance and risk management practices.
Case 1: Identifying Complex Ownership Structures
We explore a database containing over 10 million individuals and organisations, along with approximately 11 million relationships. We demonstrate how to uncover complex ownership structures involving at least five levels of relationships using Hume Advanced Expand feature, a visual query building tool. This allows us to identify matches in the database without writing queries, displaying the results directly on the screen. Traditional data structures, like tables or Excel sheets, often fail to effectively represent such intricate networks. Instead, we visualise these relationships as graphs, providing a clearer and more intuitive understanding of ownership structures.
Case 2: Familial Relationships and Grouping
Delving deeper into ownership networks, we uncover familial relationships within ownership structures. Leveraging advanced grouping techniques, we identify clusters of entities linked by familial ties, enhancing our understanding of familial wealth distribution and ownership dynamics. Through visual representations of familial groups, analysts gain clarity on interconnected relationships, facilitating more nuanced analysis and decision-making.
Case 3: Identifying Circular Ownership Structures
Circular ownerships, which involve entities owning shares in each other in a closed loop, are illegal in many countries because they can obscure true ownership, facilitate fraud, and undermine financial transparency and market integrity. They pose a unique challenge in UBO investigations due to their secretive nature. Here, we showcase how Hume’s advanced querying capabilities enable analysts to probe complex ownership paths and uncover circular ownership arrangements. By automating the detection of entities with incoming and outgoing ownership relationships, analysts can identify secretive ownership structures with ease, fostering transparency and accountability.
Case 4: Cross-Data Source Analysis
Cross-data source analysis is essential for gaining a holistic understanding of ownership networks. Here, we demonstrate how Hume facilitates the traversal of interconnected entities across diverse datasets. By leveraging entity resolution techniques, analysts can seamlessly navigate ownership paths spanning multiple data sources, uncovering hidden connections and facilitating comprehensive analysis. Through visual representations of cross-data source relationships, analysts gain valuable insights into complex ownership networks.
Case 5: Identifying Sanctioned Entities Using Entity Resolution
We focus on identifying sanctioned entities within ownership networks. We explore the challenge of unifying ownership structures across disparate datasets. Through the ingestion of Open Ownership Statements and subsets of Open Sanctions data, we highlight the importance of detecting entities sanctioned by regulatory authorities. GraphAware Hume’s entity resolution capabilities streamline the process of identifying and consolidating entities with varying attributes. By unifying ownership structures, analysts gain a comprehensive view of complex networks, enabling deeper insights into beneficial ownership relationships.
Case 6: Automation for Proactive Monitoring of Sanctioned Individuals
Finally, we explore the importance of automation for proactive insights in UBO investigations. Through Hume’s monitoring capabilities, analysts can define and automate detection patterns to identify emerging risks and trends in ownership networks. By receiving real-time alerts on newly detected patterns, analysts can stay ahead of evolving threats and take proactive measures to mitigate compliance risks. Automation empowers analysts to efficiently monitor ownership networks, ensuring robust risk management practices and regulatory compliance.
Each section showcases a distinct aspect of UBO investigations, highlighting the agility, speed, and capability facilitated by graph technology and tools like Hume. Through a combination of data integration, entity resolution, advanced querying, and proactive monitoring, analysts can unlock deeper insights into ownership networks, mitigate risks, and foster transparency in the fight against financial crime.
Conclusion
Knowledge Graph technology revolutionises UBO investigations by offering unparalleled agility, speed, and capability. From data integration and entity resolution to advanced querying and proactive monitoring, tools like Hume empower analysts to navigate complex ownership networks with confidence and precision. By harnessing the power of graphs, organisations can unlock deeper insights, mitigate risks, and foster transparency in the global fight against financial crime.
Unlocking Complex Ultimate Beneficial Ownership Investigation
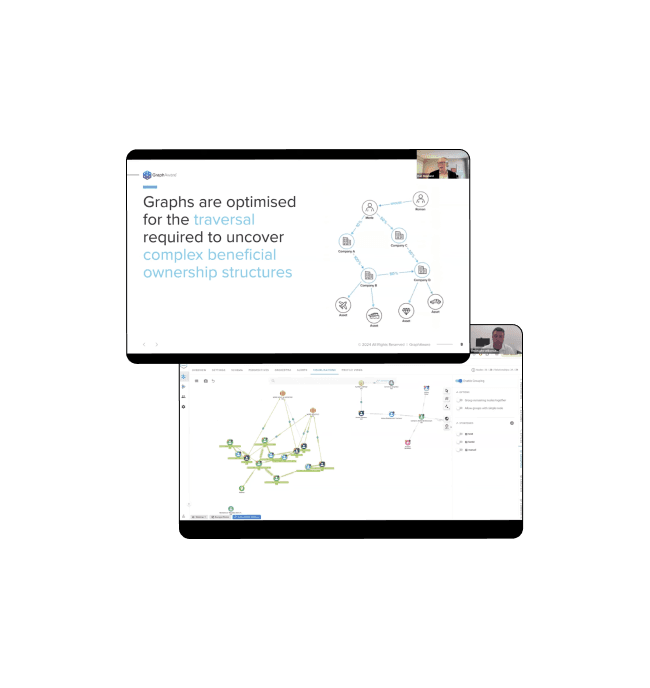